
The choice of the pulse sequence for data acquisition, the selection of an appropriate solvent suppression technique, the level of decoupling power, the type of chemical shift reference(s), the length of the 90° pulse, the number of data points collected, the repetition time, receiver gain, the quality of shimming, the quality of tuning, and the number of acquisitions will all have a significant impact on the quality of NMR spectra and the presence of peak distortions or anomalies. In addition to the sample characteristics, NMR setup and processing parameters can also have a significant impact on the quality of NMR spectra and their subsequent interpretation. These parameters include: sample pH, sample salt type and salt concentrations, dissolved oxygen content, the presence of paramagnetic ions, the choice of solvent(s), temperature, temperature gradients, spectrometer field homogeneity, and primary magnetic field strength (to name just a few). The position, intensity and spectral width of these peaks is highly dependent on the number and types of chemicals in the mixture, the corresponding spin-coupling patterns of those chemicals and a wide variety of sample parameters. In particular, the 1H NMR spectra of samples such as urine are very complex, typically consisting of > 1000 detectable and often overlapping peaks. On the other hand, NMR spectroscopy itself and the analysis of complex biological mixtures by NMR is not trivial (Tiziani et al. Furthermore, as an analytical technique NMR is robust and highly reproducible, it can be absolutely quantitative, it can be used in the precise structural determination of unknown metabolites, and it can be almost fully automated (Emwas 2015 Gonzalez-Gil et al. In particular, NMR requires: (1) little sample preparation (2) no prior chromatographic separation and (3) no chemical derivatization. 2016 Emwas and Kharbatia 2015 Emwas 2015), NMR spectroscopy has several advantages. Despite not being quite as sensitive as MS-based metabolomics (Grison et al. Now samples as small as 50 µL are being handled and nanomolar concentrations are now detectable. 2010) have significantly improved the sensitivity of NMR for metabolomics applications. 2015 Grimes and O’Connell 2011) and dynamic nuclear polarization (Emwas et al. 2002), microprobe design advances (Miao et al. 2013), cryogenically cooled probe technology (Keun et al. Continuing improvements in NMR technology, such as increased magnet field strength (> 1 GHz) (Cousin et al. Even today there are more than 600 papers published each year that describe the use of NMR in metabolomics studies. Indeed, the very first metabolomics papers were based on NMR spectral analysis of biofluids, such as urine (Serkova et al. NMR has played an important role in the development and the continuing advances in metabolomics over the past two decades. We also provide a set of recommendations for best practices regarding spectral and data processing for NMR-based metabolomic studies of biofluids, with a particular focus on urine. These include: chemical shift referencing, phase and baseline correction, spectral alignment, spectral binning, scaling and normalization.
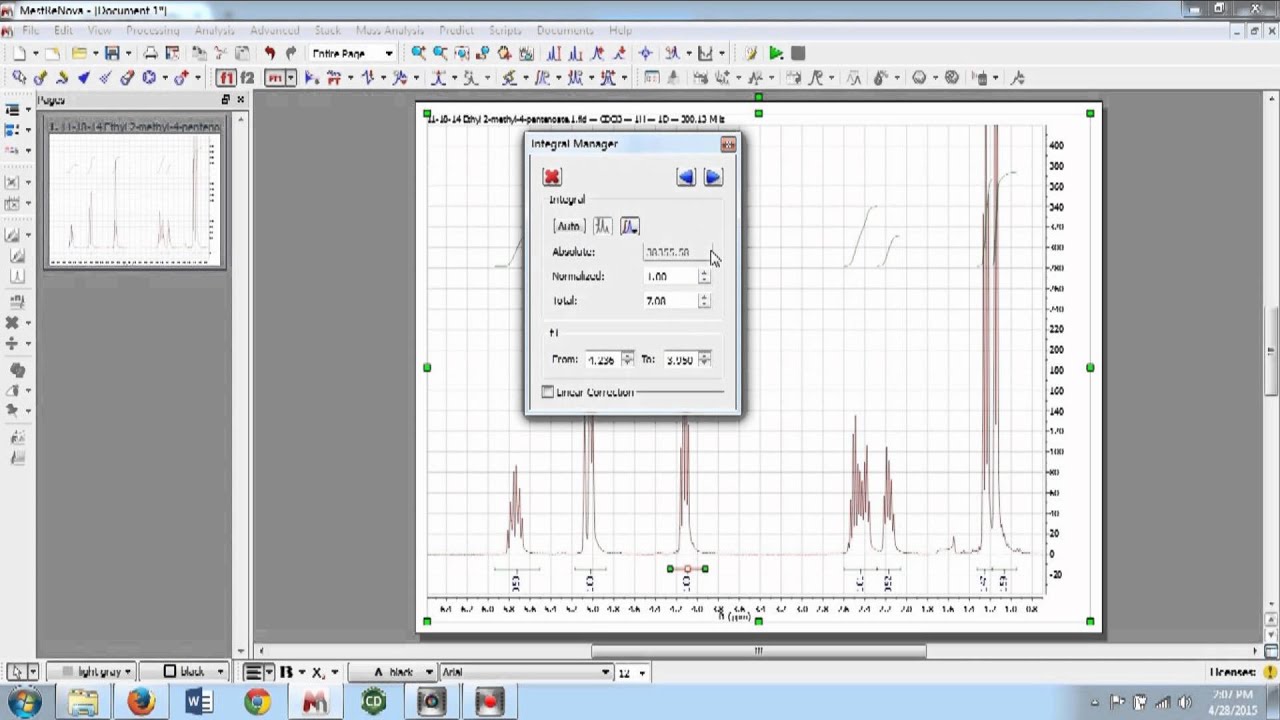
In this review we highlight the significance, advantages and disadvantages of different NMR spectral processing steps that are common to most NMR-based metabolomic studies of urine. Therefore, proper processing of NMR data is a critical step to correctly extract useful information in any NMR-based metabolomic study. As a result, a number of data processing techniques such as scaling, transformation and normalization are often required to address these issues. For instance, signals originating from the most abundant metabolites may prove to be the least biologically relevant while signals arising from the least abundant metabolites may prove to be the most important but hardest to accurately and precisely measure.

Furthermore, because NMR permits the measurement of concentrations spanning up to five orders of magnitude, several problems can arise with data analysis. If the NMR spectra are mis-phased or if the baseline correction is flawed, the estimated concentrations of many compounds will be systematically biased. For instance, if the NMR spectra are incorrectly referenced or inconsistently aligned, the identification of many compounds will be incorrect. However, the quality and utility of these insights can be profoundly affected by how the NMR spectra are processed and interpreted. 1H NMR spectra from urine can yield information-rich data sets that offer important insights into many biological and biochemical phenomena.
